Prediction as a Service (PraaS)
Harness the power of AI and ML to drive predictive outcomes and propel your business.
• Lower the barrier of entry to AI
• Reduce the time to value
• Proveable Performance
• No Need for in-house Data Science
Solutions
Our Prediction as a Service (PraaS) solution framework is structured to validate and quantify ROI of a potential use case, assess model performance and the feasibility of integrating within a company's infrastructure before the customer makes a commitment to production development.
Once a production model is developed and deployed, we continue to manage the deployment, assessing the performance over time, and comparing different models to deliver the best possible performance.
Problems we help solve
Customer Churn
Product Demand Forecast
Workforce Demand Forecast
Financial Forecast
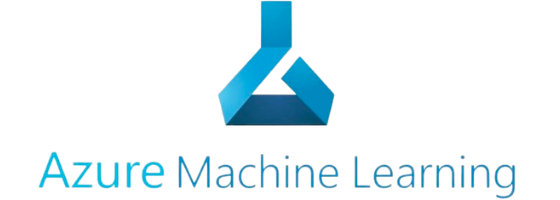
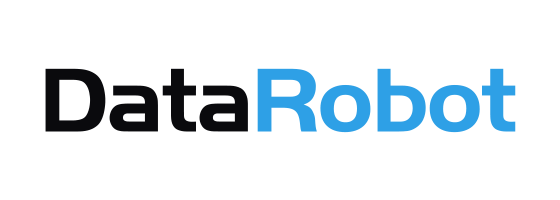
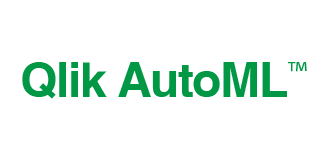
Case Study
Companies today face a dilemma, realising the need for AI adoption to stay relevant in the long term, while being presented with heavy investment decisions to implement prior to proving value.
Whether heavy investment in a platform or Data Science team, the time and financial cost of entry is often huge.
This high barrier to entry is causing companies to stall the development of this critical capability, and the costs down the road will be huge.
With Data Technology's Prediction as a Service, we remove the need for in-house Data Science teams, and through our feasibility studies we give our clients the confidence of success before committing to the development and management of a production model.