Translating Business Problems to Machine Learning Solutions: A Guide to Understanding and Maximizing the Benefits
Machine Learning is rapidly becoming a crucial technology in the business world, with thousands of companies around the globe leveraging predictive analytics to increase revenue growth and improve efficiency. However, many organizations do not fully understand the importance of collaboration between business and technical experts in order to build a Machine Learning solution that truly delivers value. This guide will provide an overview of the key steps businesses should take in order to successfully translate business problems into effective Machine Learning solutions.
Step 1: Identifying Use Cases
The first step in translating business problems into Machine Learning solutions is to identify potential use cases. This involves examining the processes behind a company's key performance indicators (KPIs) to identify areas where Machine Learning could be used to improve performance. For example, a company's retention team might aim to keep customers purchasing from the company, and could use a predictive model to determine which customers are at risk of leaving. By working closely with business experts, data scientists can gain an understanding of which information is most valuable and which problems the Machine Learning solution should aim to solve.
Step 2: Understanding the Problem
Once a potential use case has been identified, it is important for both business and technical experts to work together to fully understand the problem they are trying to solve. This involved gathering the data on the current process, and assessing the quality of this data both for its completeness and accuracy. It is also important to consider factors that may be driving customer behaviour or process outcomes, and to identify any gaps in knowledge that may need to be filled.
Step 3: Evaluating Timing and Availability of Predictions
One of the key considerations in developing a Machine Learning solution is timing. When do you need the predictions to be made? Timing is critical for any business process, and it is essential to make predictions with enough time for the business to act. The timing of predictions will also influence the criteria that the models must be scored on, and it is important to answer this question as soon as possible when developing a solution.
Step 4: Understanding Business Knowledge and Domain Expertise
Data scientists are experts in the underlying algorithms, statistics, and mathematics that make up Machine Learning. However, they often lack the domain knowledge needed to get the best results in use cases related to the business. Business experts, on the other hand, have a deep understanding of the factors that affect customer behaviour or process outcomes. It is essential for business experts to communicate with data scientists early on to ensure that the data set used to train the model accurately represents the factors that drive the outcome of interest.
Step 5: Implementing the Model and Integrating Predictions into Business Processes
Once a Machine Learning model has been developed, it is important to ensure that the predictions made by the model are used to improve business processes. This may involve batch processing predictions on a regular time schedule, integrating predictions into existing software systems, or making predictions in real-time. It is also important to consider how the end user will access the predictions and how the predictions will be communicated to stakeholders.
Step 6: Monitoring and Improving the Model
The final step in translating business problems into Machine Learning solutions is to monitor and improve the model. This involves regularly monitoring the accuracy of the model and adjusting the model as needed to ensure that it continues to deliver value. It is also important to continually evaluate the business processes in which the model is used, and to make changes as needed to improve performance.
In conclusion, translating business problems into Machine Learning solutions is a complex process that requires collaboration between business and technical experts. By following the steps outlined in this guide, businesses can ensure that they are able to build solutions that deliver real value.
If you would like to learn more about how your business can use AI to drive ROI, please join Matthew Sumner at 11:00am on the 18th of March for his complimentary webcast on the topic.
Share this
You May Also Like
These Related Stories
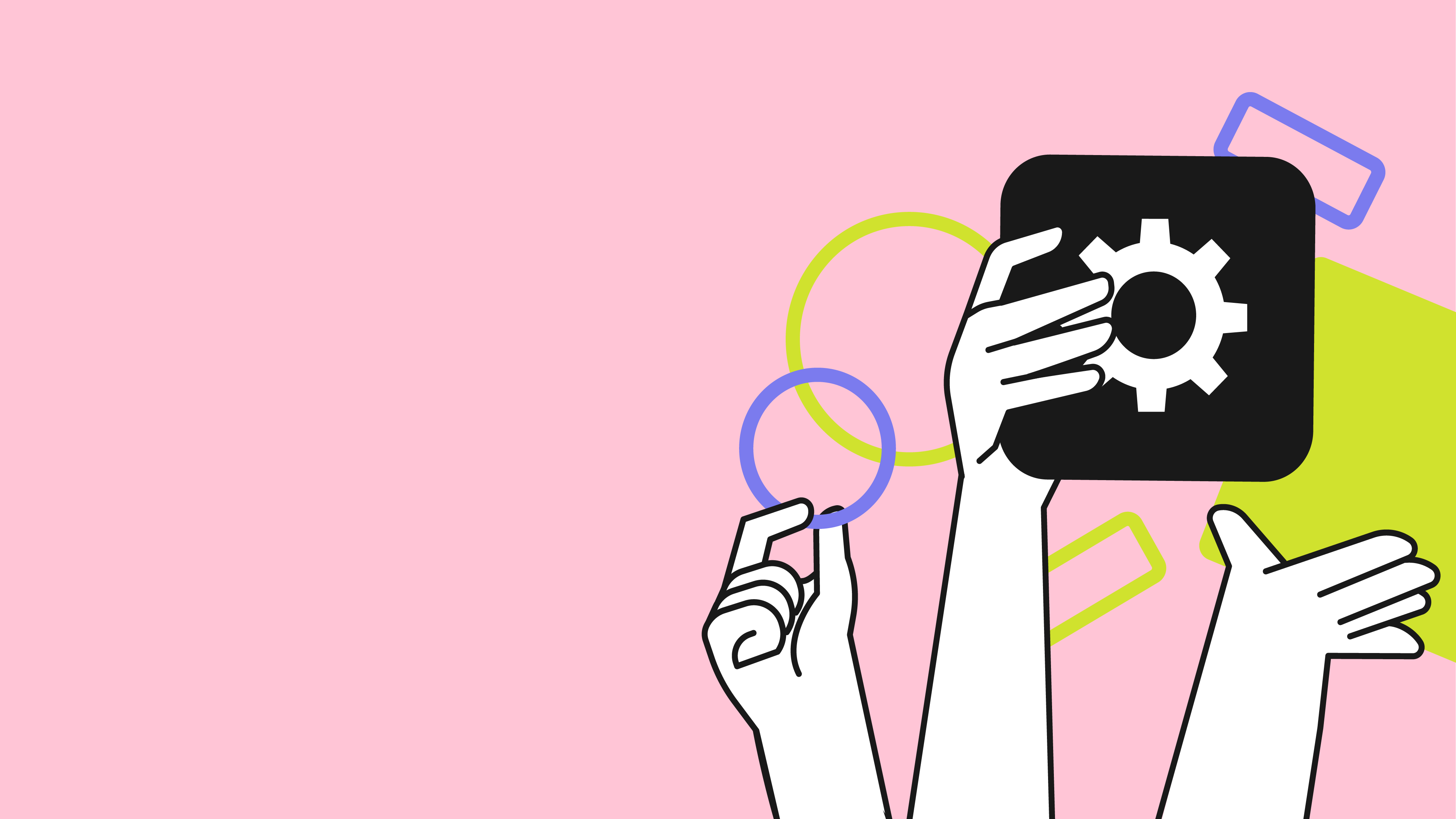
Your Workforce, Powered by Machine Learning
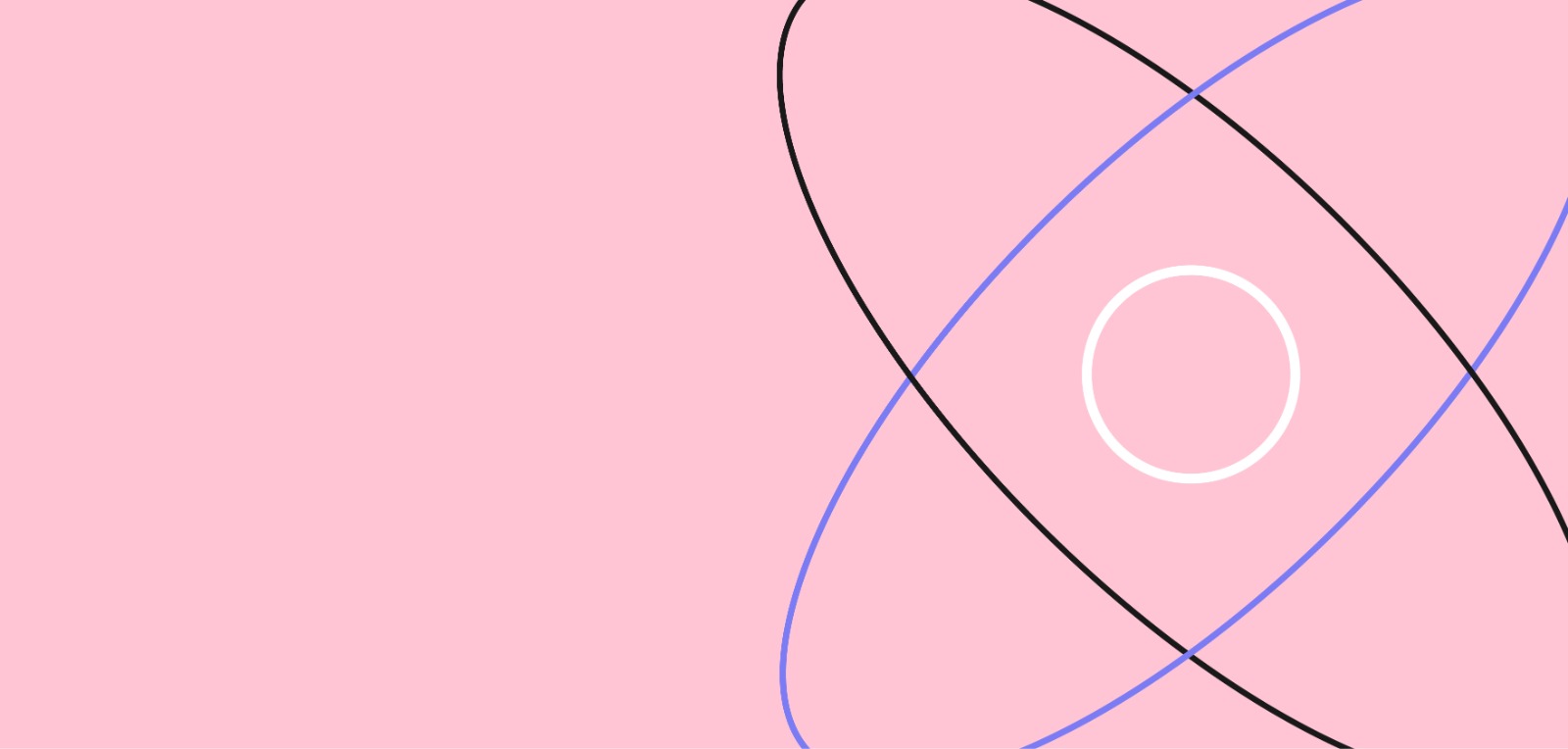
5 Considerations for Machine Learning Mastery
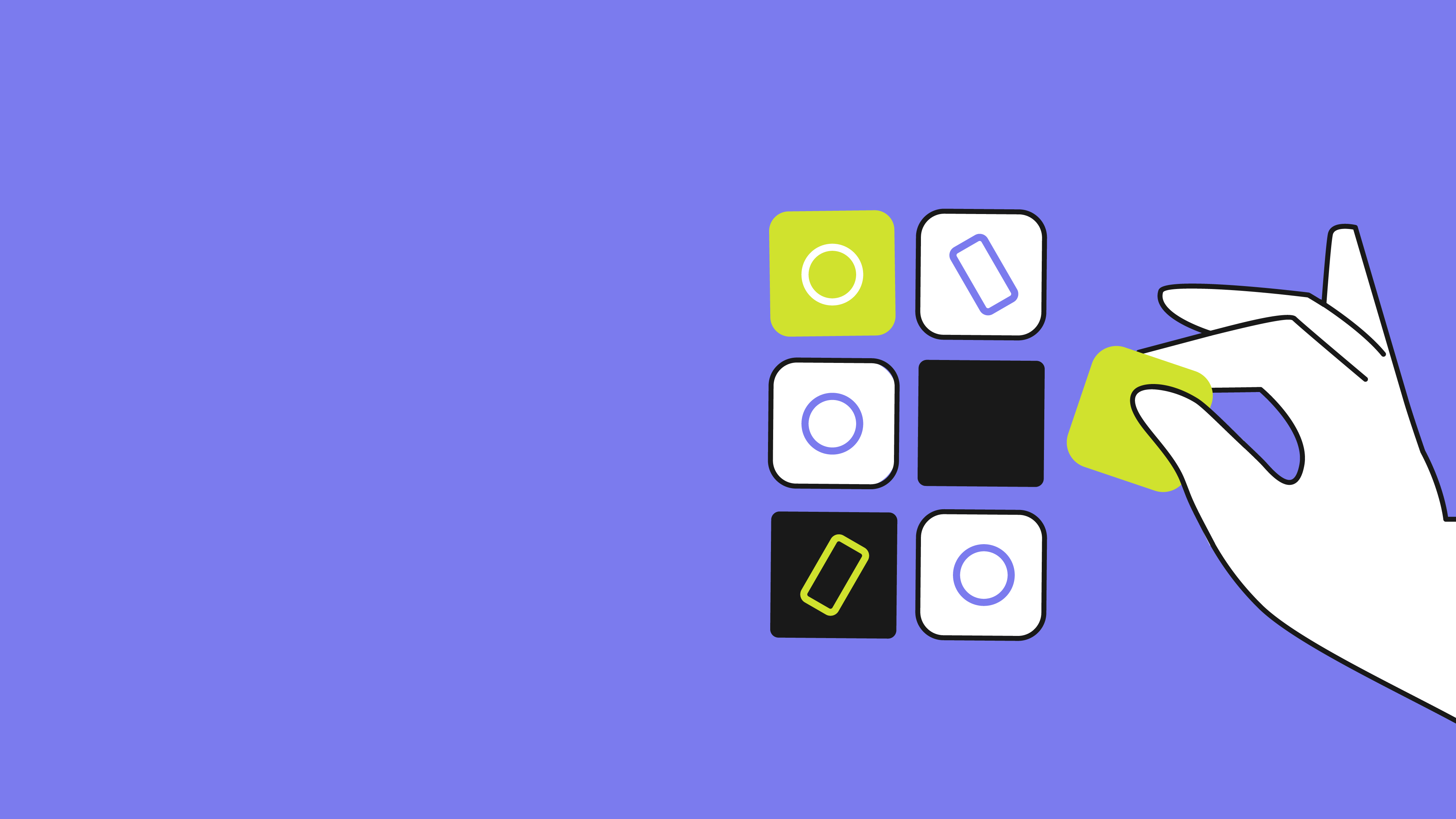